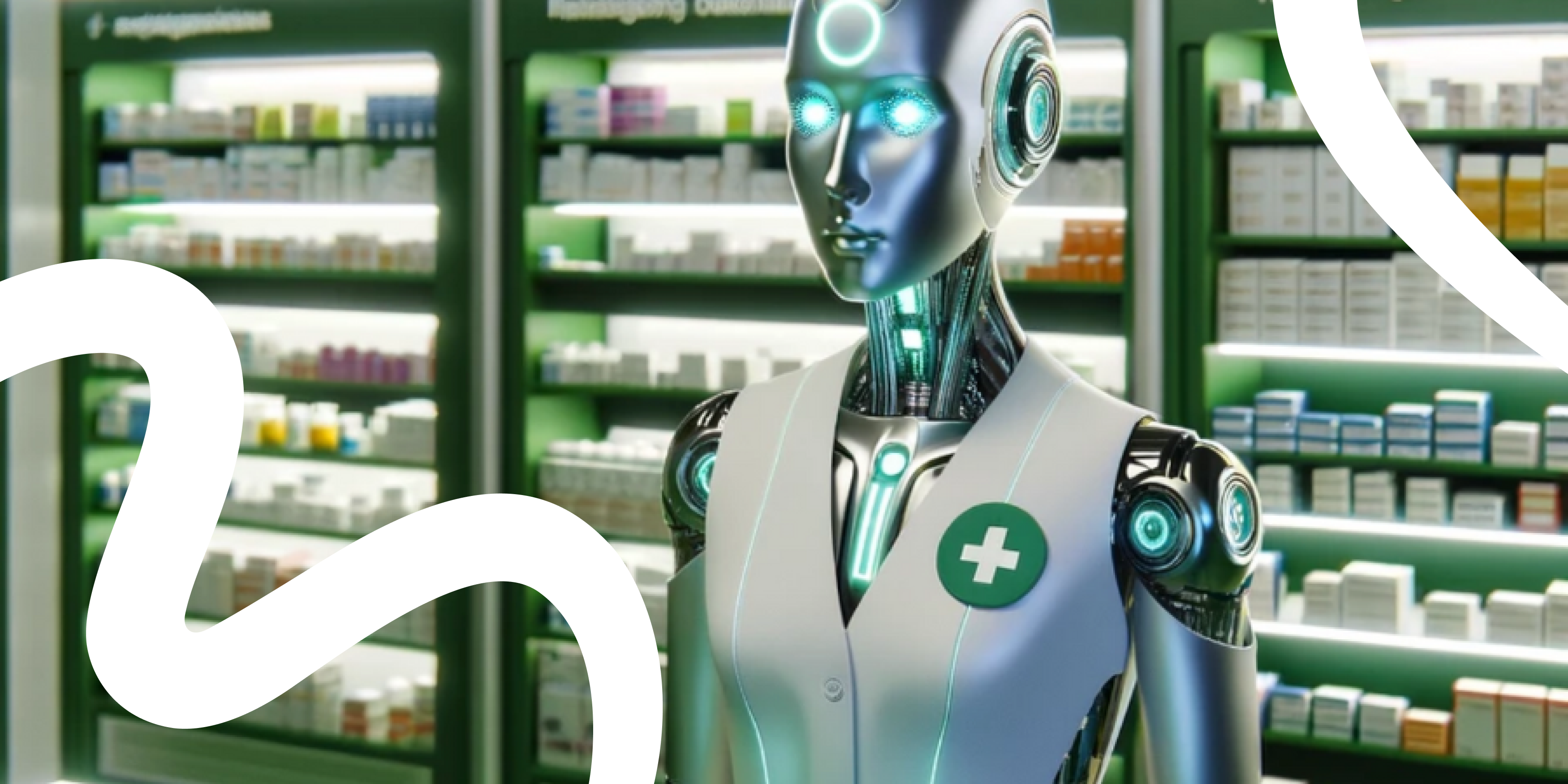
Generative AI in Healthcare: Insights from Apoteket Collaboration
-
Leo Nathorst-Böös
This fall, we embarked on a generative AI journey with our partner, Apoteket, Sweden’s largest pharmaceutical retailer. Deep-diving in prompt engineering, autonomous agents, and conversational design, we set out to prototype the virtual pharmacist of the future.
This past year, I’ve been thinking a lot about what it is that excites me about new technology. Apart from the obvious excitement of making me feel like I’m living at the beginning of an Isaac Asimov novel, new platforms and tools allow us to solve old problems in new ways. New innovations get us thinking about the stuff that we haven’t been able to figure out before, and they provide new ways forward with those annoying user problems we’ve been acutely aware of but still haven’t fixed. In this article, I’ll share four key insights and unexpected lessons from our adventure with Apoteket. While we didn’t need to teach our pharmacist Hilda Asimov’s three laws of robotics, we did learn to give her some guardrails. But more on that further down.
Enter: Apoteket
Over the past year, we, like the rest of the world, have been smitten by AI's possibilities. Since APIs for using LLMs from OpenAI and Google have become available, more of our partners have been interested in seeing how generative AI can improve their businesses. We’ve also built some internal tools, improved our services, and tried out new ideas. And we’ve been having a lot of fun.
With Apoteket, we partnered up on a project to explore how generative AI can help them help customers find the right advice and products. With a huge product catalog and diverse customer needs, we set out to replicate the experience of talking to a skilled pharmacist at one of their retail locations. Friendly, understanding, helpful, and very knowledgeable. But also completely safe. When recommending products for health and self-care, we can’t afford hallucinations from our tech; we need to know that the answer our customers get is correct.
Since this is a new field for everyone, we opted to learn together with Apoteket, and that was our most important success metric for the project: how much we learned. No one is an expert on these platforms yet, so we wanted to enter with an open mind and see where it would take us. Rather than create the best possible product for end-users—which is usually part of our mission at Bontouch—we spent more time on our learnings than the product itself and had a very open weekly check-in where we discussed our recent findings and how to proceed next.
When you’re working with cutting-edge technologies, it’s good to keep your eyes focused on the vision for your product and what you want to learn. If you’re too inflexible about the tech solution, you’ll quickly run into problems because things change very fast. And that takes us to our first learning.
Learning 1: Be ready to pivot - your first idea is unlikely to be your best one
When we started working on the Hilda project with Apoteket, Chat GPT Plugins was the latest and greatest offering from Open AI. The idea to build a product that users can interact with in the Open AI interface excited us, with all that it would mean for the user experience design and use of their latest tech. But we quickly ran into a wall: the plug-in we would do would not be production-ready as part of this project. To test it privately, anyone who wanted to try it needed to be an enrolled ChatGPT developer. We had one account. And OpenAI was not rolling plug-in development out to more people. We later learned why—they were working on the much better “GPTs”—but we didn’t know that then. This was not going to work.
We weren’t married to the tech solution, so we quickly started exploring other ways of solving the problem. When we presented an alternative solution to Apoteket, we realized this would be even better than what we first had envisioned. Running into a developer account enrollment wall and having to pivot turned out to be great for the project.
Our second idea was a prototype landing page mimicking apoteket.se with a chat interface integrated into the site, using the OpenAI APIs instead of a plug-in. This would create a more controlled, easily discoverable experience for web visitors and an opportunity to explore other technologies as well. The team at Apoteket liked it, and we got to work.
Learning 2: Chat GPT is not a trained pharmacist
Medical advice is special. But it’s not the only time you want to ensure that you get the exact right information from your services. LLMs have a tendency to hallucinate in their answers. And while they’re getting better all the time, being right 99% of the time wouldn’t cut it. We needed to be perfect.
To solve this, we decided to use ChatGPT for some parts and a traditional backend service for other parts. ChatGPT is great at understanding the user’s natural language and transforming it into structured data. It can also take the prepared data from the backend and “humanize” it to feel more like a conversation. This gave us the best of both worlds: a natural, human-like conversation with the user and quality-controlled medical advice that the user can trust.
Learning 3: Large language models aren't big brains that can do anything
This is the point where I got confused. Luckily, I had some really smart people by my side who could teach me. While this is more a reflection of my ignorance than a big learning for the team, I suspect others are still as naive as I am when it comes to understanding how these new technologies actually work.
When we were designing Hilda, our virtual pharmacist, I was imagining that what we would do would be to provide a set of instructions to the LLM, along with unstructured data that it would need, and then it would sort of just… figure it out. I know how stupid this sounds. But this is what we do to humans, right? Here’s a book. It contains the information you need to learn. Read it, and then we’ll test you on it. Oh, and here’s a list of products. There are some keywords in there, so you can match up the products with what’s in the book. Good luck! Nope. That’s not what we do with LLMs. At least not when we want it to be exact. We need traditional things like backend services and APIs. And databases. The future disappoints again!
Learning 4: Guardrails make all the difference
After a few weeks of work, it was time to put our work to the test. We had a frontend chat interface that asked you what you needed help with and, with the help of our backend, turned that into tailored product recommendations and advice. We had told ChatGPT it was a pharmacist giving advice and that it should be friendly and understanding. We hadn’t told it it was called Hilda, so that got pretty confusing at first when users said Hello, Hilda. But more importantly, we hadn’t realized people may be telling it about serious medical conditions. So regardless of whether you told Hilda about a mild headache or that you were experiencing a heart attack, it responded in the same way: I understand that this is a tough situation for you, but I assure you this is a common issue and nothing to be worried about. I’m here to help. Oops.
We were clearly missing some very important “guardrails.” Guardrails in AI are guidelines and boundaries ensuring that the service doesn’t do anything it’s not supposed to do. You may have experienced a guardrail when talking to Chat GPT. If you don’t know what I’m talking about, ask it to say something mean to you, and you’ll see that it won’t: I won't say anything mean. That’s guardrails at work. For Hilda, we needed guardrails to ensure it couldn’t give any advice we hadn’t explicitly provided. It had to recommend the user speak to a medical professional if they were asking about serious medical conditions. And many others. Asimov would be proud.
Final remarks
Our twelve weeks went by fast. At the end of the project, we were satisfied that we had created a proof of concept that could show Apoteket how to continue these explorations in-house. We learned a lot together, just as we set out to do, and every AI project we’ve done since continues to build on these learnings.
As I said at the beginning of this article, the way technology provides new ways of solving old problems is what excites me the most about working in an innovative field. With the speed at which generative AI developments are moving, new tools are coming out all the time. GPTs, released just at the end of this short project, could very well have been an even better tool than what we went for. And I’m sure there will be another one that will be even better soon. With some luck, I’ll have my big brain soon, and we won’t need as much structure. Just a teacher, some books, and a well-written test. Just like we do when teaching humans. A product manager can dream, right?
Want to explore these future possibilities with us? Don’t hesitate to get in touch. We’d love to put our skills to the test for your business and learn together.